Commentary | Open Access | Volume 4 | Issue 1 | 2023 | DOI No.: 10.46439/stemcell.4.018
The tumor dose sensitivity matrix and stem cells in head and neck cancer
George D Wilson1,2,*, Di Yan1,3
1Department of Radiation Oncology, Corewell Health, Royal Oak, Michigan, USA
2Henry Ford Health, Detroit, Michigan, USA
3Department of Radiation Oncology, West China Hospital, Sichuan University, China
*Corresponding Author:
George D Wilson
Department of Radiation Oncology,
Corewell Health,
Royal Oak, Michigan, USA
E-mail: george.wilson@corewellhealth.org
Received date: October 02, 2023; Accepted date: October 13, 2023
Citation: Wilson GD, Yan D. The tumor dose sensitivity matrix and stem cells in head and neck cancer. Arch Stem Cell Ther. 2023;4(1):1-4.
Copyright: © 2023 Wilson GD, et al. This is an open-access article distributed under the terms of the Creative Commons Attribution License, which permits unrestricted use, distribution, and reproduction in any medium, provided the original author and source are credited.
The Problem of Delivering Curative Doses of Radiation Therapy
Radiation therapy (RT) is one of the pillars of locally advanced head and neck cancer (HNSCC) treatment in combination with cisplatin or epidermal growth factor receptor inhibitors. Despite a very high local tumor control rate, approximately 50% of patients with locally advanced disease will develop a recurrence [1]. Although the human papilloma virus (HPV)-associated subtype of HNSCC generally have a better prognosis even they may recur even after standard chemoradiation. This has ramifications for the current trend to de-intensify therapy for HPV-associated oropharyngeal HNSCC with an aim of reducing treatment-associated morbidity and side effects [2]. However, outside of clinical trials patients with HPV-positive and negative tumors receive similar RT treatment uniformly prescribed to the whole tumor. Studying geographic patterns of failure after radiotherapy can aid in determining the optimal dose and distribution of RT in different patient subgroups [3] but even more attractive would be the ability to answer the fundamental question of how much radiation needs to be given to each individual patient and how much dose needs to be delivered to specific sub-regions of each tumor. It is well known that human tumors of the same or different histology, stage and site vary considerably in their radiation dose response. Thus, the ability to detect and predict inter- and intra-tumoral dose sensitivities would have significant impact on the clinical practice of radiotherapy [4].
The Tumor Dose Sensitivity Matrix: Does it Identify Cancer Stem Cells?
We have previously reported the development of a tumor dose sensitivity or response matrix (DRM) [5,[6] that was constructed at the positron emission tomography (PET) image voxel-level using multiple fluorodeoxyglucose (FDG)-PET/computed tomography (CT) images obtained before and during the early weeks of chemoradiation for HNSCC. The tumor voxel dose sensitivity, DRM, was derived based on the first order reaction law of cell killing from the linear regression of logarithmic ratio of tumor metabolic activity with treatment and is unique for each voxel. The tumor dose sensitivity matrix is highly individualized and has a strong capability to identify radioresistant tumor voxels during the early course of radiotherapy. Combining with the pre-treatment baseline PET standardized uptake vale (SUV), it results in a high capability to predict local tumor failure (Figure 1). Importantly, a tumor voxel control probability (TVCP) lookup table was created using the maximum likelihood estimation on these key tumor voxel parameters, the tumor voxel DRM and baseline SUV, which can be used as the objective function for adaptive dose painting by numbers (DPbN)-based inverse planning optimization [5,6]. In addition, considering the efficacy of hypofractionation for resistant tumor cells, early identification of distinct subpopulations derived from the intratumoral dose response could also be utilized to design dose fractionation painting by numbers (DFPbN) adaptively.
Figure 1. The vertical columns show the pre-treatment FDG-PET images (upper), post-treatment FDG-PET images at local recurrence (center) and DRM images during treatment after 40 Gy (lower) for the four patients that failed treatment locally. The green circle represents the same tumor voxels in each image.
The response of tumors to radiation can be modified by many biological activities including tumor cell metabolism, hypoxia, reoxygenation, proliferation, vascular perfusion, immune cells, cancer associated fibroblasts and other components of the tumor microenvironment. All the major tumor biological hallmarks have been proposed to directly or indirectly connect to response to radiation [7]. The approach described above is unique in that it has the ability to describe an intra-tumoral dose sensitivity distribution using dynamic changes in the whole tumor in situ during treatment where many of the aforementioned features will be dynamically changing. This sets it apart from other studies that have developed a radiosensitivity index (RSI) based on biopsies [8]. The RSI has subsequently been validated in several independent clinical datasets [9-12]. Importantly, unlike many other studies where the identification of radioresistant tumors had no direct pathway to prescribe alternative treatment options, the RSI has been combined with linear quadratic modeling to develop the concept of the genomic-adjusted radiation dose (GARD) [13]. Similar to the gold standard clonogenic assay [14,15], this molecular assay provides only a single averaged parameter for each human tumor and does not take into account the microenvironment of the human tumor. Therefore, it cannot identify the intra-tumoral heterogeneity of dose response for individual human tumors.
Subsequently, we undertook a study on the pretreatment biopsies from the patients who had undergone the multiple imaging studies to see if there was any correlation with parameters derived from the tumor dose sensitivity matrix and some selected major biological indices. One of the most interesting findings of the study was the positive and highly significant correlation between CD44 expression and volume of tumor with a DRM greater than 0.8; this is indicative of a high degree of radioresistance. CD44-positive HNSCC cells have been previously shown to initiate tumor growth in immunocompromised animals much more efficiently than CD44-negative cells. This indicates that cancer stem cells (CSCs) are enriched in the CD44-positive subpopulation of HNSCC [16] and have been shown by [17,18] to predict local control after radiotherapy in HNSCC. Our data showed that specific foci of cells are responsible for tumor recurrence based on the imaging which is consistent with concept that CSCs may be randomly distributed in tumors in specific niches [19].
Cancer Stem Cells and Tumor Metabolism
In recent years evidence has accumulated showing that there are multiple genetically diverse clones that co-exist within various kinds of tumors and that CSCs are a distinct subpopulation within a tumor [20]. Not all cells within a tumor are equally sensitive to RT such that understanding the diverse radiosensitivity of different tumor cell subpopulations will be necessary to optimize RT. This challenges the common practice of employing macroscopic bulk tumor responses using medical imaging as the primary endpoint for determining the effectiveness of an anti-neoplastic treatment. Indeed, in our study, there was no correlation between the SUV obtained before and during treatment and the tumors that failed RT [5]. It would seem unlikely that the overall tumor response represents the response of all cells within the tumor, including the most resistant subpopulation within the tumor. The stem cell model of cancer may explain the existence of discrete subpopulations of cells with different genetic and phenotypical differences that set them apart from the bulk of the tumor and equip them to resist treatments and cause local recurrences.
It has been recognized that CSCs are able to self-renew and differentiate and possess a high capability to repair DNA damage, exhibit low levels of reactive oxygen species (ROS), and proliferate slowly. These features tend to render CSC resistant to various therapies, including radiation therapy (RT). More recently, it has been documented that CSCs have different metabolic phenotypes compared with differentiated cancer cells. CSCs can dynamically transform their metabolic state to favor glycolysis or oxidative metabolism whilst most cancer cells use the less efficient glycolysis to produce ATP and essential biomolecules [21-24]. The typical glycolytic pathway includes several enzyme reactions. The first is the phosphorylation of glucose to glucose-6-phosphate, a process catalyzed by hexokinase (HK). The high expression and activity of hexokinase 2 (HK2) in cancer cells is the basis to detect and image tumors by FDG PET-CT. This feature of tumors has established an important role Indeed for FDG PET–CT in the staging and early detection of disease recurrence in number of solid tumors. It is thought that FDG PET–CT is a useful metabolic imaging modality that can measure glucose uptake and potentially reflect tumor aggressiveness [25]. However, the role of FDG PET-CT in predicting intra-tumoral dose response or local tumor niches that are resistant to radiation dose is not well-established [26-30].
Our data is consistent with heterogeneity and plasticity within the stem cell compartment. Those tumors that failed did not show any clear patterns on tumor voxel dose sensitivity distribution. Resistant tumor voxels were not correlated to the pre-treatment SUV and not at any special location within tumor. Based on the post-treatment PET-CT images, the recurrent tumor voxels were among the resistant voxels. However, large numbers of tumor voxels for these 4 failures were within the controlled region and some of them were very sensitive to the treatment dose. For these tumor voxels, a treatment dose, even lower than the standard prescription dose of 70Gy, could be appropriate. This highlights the variation in radiosensitivity and offers the possibility to escalate and de-escalate treatment plans based on individual voxel sensitivity.
In summary, the imaging method we have developed is a unique approach to study the response to radiation therapy of the whole tumor at the level of the individual voxel. The dynamic metabolic changes we have observed will be influenced by the tumor microenvironment and other heterogeneous physiological changes and biological variation that exist in the tumor before and during the early part of treatment. The relationship between the resistant tumor voxels and CSCs requires further study but the method already presents the opportunity to design dose and fractionation schedules that can deliver tailored treatment based on the expected radiosensitivity of the tumor voxel.
Funding
This research was supported by Departmental funds.
References
- Rettig EM, D’Souza G. Epidemiology of head and neck cancer. Surgical Oncology Clinics. 2015 Jul 1;24(3):379-96.
- Mirghani H, Amen F, Blanchard P, Moreau F, Guigay J, Hartl DM, et al. Treatment de‐escalation in HPV‐positive oropharyngeal carcinoma: ongoing trials, critical issues and perspectives. International Journal of Cancer. 2015 Apr;136(7):1494-503.
- Nissi L, Suilamo S, Kytö E, Vaittinen S, Irjala H, Minn H. Recurrence of head and neck squamous cell carcinoma in relation to high-risk treatment volume. Clinical and Translational Radiation Oncology. 2021 Mar 1;27:139-46.
- Suit H, Skates S, Taghian A, Okunieff P, Efird JT. Clinical implications of heterogeneity of tumor response to radiation therapy. Radiotherapy and Oncology. 1992 Dec 1;25(4):251-60.
- Yan D, Chen S, Krauss DJ, Deraniyagala R, Chen P, Ye H, et al. Inter/intra-tumoral dose response variations assessed using FDG-PET/CT feedback images: Impact on tumor control and treatment dose prescription. Radiotherapy and Oncology. 2021 Jan 1;154:235-42.
- Yan D, Chen S, Krauss DJ, Chen PY, Chinnaiyan P, Wilson GD. Tumor voxel dose-response matrix and dose prescription function derived using 18F-FDG PET/CT images for adaptive dose painting by number. International Journal of Radiation Oncology* Biology* Physics. 2019 May 1;104(1):207-18.
- Good JS, Harrington KJ. The hallmarks of cancer and the radiation oncologist: updating the 5Rs of radiobiology. Clinical Oncology. 2013 Oct 1;25(10):569-77.
- Torres-Roca JF, Eschrich S, Zhao H, Bloom G, Sung J, McCarthy S, et al. Prediction of radiation sensitivity using a gene expression classifier. Cancer Research. 2005 Aug 15;65(16):7169-76.
- Ahmed KA, Chinnaiyan P, Fulp WJ, Eschrich S, Torres-Roca JF, Caudell JJ. The radiosensitivity index predicts for overall survival in glioblastoma. Oncotarget. 2015 Oct 10;6(33):34414-22.
- Eschrich SA, Fulp WJ, Pawitan Y, Foekens JA, Smid M, Martens JW, et al. Validation of a radiosensitivity molecular signature in breast cancer. Clinical Cancer Research: An Official Journal of the American Association for Cancer Research. 2012 Sep 15;18(18):5134-43.
- Strom T, Hoffe SE, Fulp W, Frakes J, Coppola D, Springett GM, et al. Radiosensitivity index predicts for survival with adjuvant radiation in resectable pancreatic cancer. Radiotherapy and Oncology. 2015 Oct 1;117(1):159-64.
- Torres-Roca JF, Fulp WJ, Caudell JJ, Servant N, Bollet MA, Van De Vijver M, et al. Integration of a radiosensitivity molecular signature into the assessment of local recurrence risk in breast cancer. International Journal of Radiation Oncology* Biology* Physics. 2015 Nov 1;93(3):631-8.
- Scott JG, Berglund A, Schell MJ, Mihaylov I, Fulp WJ, Yue B, et al. A genome-based model for adjusting radiotherapy dose (GARD): a retrospective, cohort-based study. The Lancet Oncology. 2017 Feb 1;18(2):202-11.
- Björk-Eriksson T, West C, Karlsson E, Mercke C. Tumor radiosensitivity (SF2) is a prognostic factor for local control in head and neck cancers. International Journal of Radiation Oncology* Biology* Physics. 2000 Jan 1;46(1):13-9.
- West CM, Davidson SE, Roberts SA, Hunter RD. Intrinsic radiosensitivity and prediction of patient response to radiotherapy for carcinoma of the cervix. British Journal of Cancer. 1993 Oct;68(4):819-23.
- Prince ME, Sivanandan R, Kaczorowski A, Wolf GT, Kaplan MJ, Dalerba P, et al. Identification of a subpopulation of cells with cancer stem cell properties in head and neck squamous cell carcinoma. Proceedings of the National Academy of Sciences. 2007 Jan 16;104(3):973-8.
- Baschnagel AM, Tonlaar N, Eskandari M, Kumar T, Williams L, Hanna A, et al. Combined CD 44, c‐MET, and EGFR expression in p16‐positive and p16‐negative head and neck squamous cell carcinomas. Journal of Oral Pathology & Medicine. 2017 Mar;46(3):208-13.
- de Jong MC, Pramana J, van der Wal JE, Lacko M, Peutz-Kootstra CJ, de Jong JM, et al. CD44 expression predicts local recurrence after radiotherapy in larynx cancer. Clinical Cancer Research: An Official Journal of the American Association for Cancer Research. 2010 Nov 1;16(21):5329-38.
- Baumann M, Krause M, Hill R. Exploring the role of cancer stem cells in radioresistance. Nature Reviews Cancer. 2008 Jul;8(7):545-54.
- Prasetyanti PR, Medema JP. Intra-tumor heterogeneity from a cancer stem cell perspective. Molecular Cancer. 2017 Dec;16(1):41.
- Trosko JE. Evolution of energy metabolism, stem cells and cancer stem cells: how the Warburg and Barker hypothesis might be linked. BMC Proceedings. 2013 Apr 4; 7(Suppl 2):K8.
- Zhu X, Chen HH, Gao CY, Zhang XX, Jiang JX, Zhang Y, et al. Energy metabolism in cancer stem cells. World Journal of Stem Cells. 2020 Jun 6;12(6):448-61.
- Peixoto J, Lima J. Metabolic traits of cancer stem cells. Disease Models & Mechanisms. 2018 Aug 1;11(8):dmm033464.
- Yadav UP, Singh T, Kumar P, Sharma P, Kaur H, Sharma S, et al. Metabolic adaptations in cancer stem cells. Frontiers in Oncology. 2020 Jun 25;10:1010.
- Paidpally V, Chirindel A, Lam S, Agrawal N, Quon H, Subramaniam RM. FDG-PET/CT imaging biomarkers in head and neck squamous cell carcinoma. Imaging in Medicine. 2012 Dec;4(6):633-47.
- Brun E, Kjellén E, Tennvall J, Ohlsson T, Sandell A, Perfekt R, et al. FDG PET studies during treatment: prediction of therapy outcome in head and neck squamous cell carcinoma. Head & Neck. 2002 Feb;24(2):127-35.
- Castaldi P, Rufini V, Bussu F, Miccichè F, Dinapoli N, Autorino R, et al. Can “early” and “late” 18F-FDG PET–CT be used as prognostic factors for the clinical outcome of patients with locally advanced head and neck cancer treated with radio-chemotherapy?. Radiotherapy and Oncology. 2012 Apr 1;103(1):63-8.
- Hautzel H, Müller-Gärtner HW. Early changes in fluorine-18-FDG uptake during radiotherapy. Journal of Nuclear Medicine. 1997 Sep 1;38(9):1384-6.
- Hunter GJ, Hamberg LM, Choi N, Jain RK, McCloud T, Fischman AJ. Dynamic T1-weighted magnetic resonance imaging and positron emission tomography in patients with lung cancer: correlating vascular physiology with glucose metabolism. Clinical Cancer Research: An Official Journal of the American Association for Cancer Research. 1998 Apr 1;4(4):949-55.
- Juweid ME, Cheson BD. Positron-emission tomography and assessment of cancer therapy. New England Journal of Medicine. 2006 Feb 2;354(5):496-507.
Editorial Board
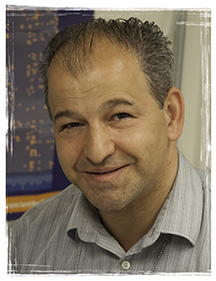
Kartoosh Heydari
UC Berkeley
USA

Giovannino Silvestri
University of Maryland School of Medicine
USA

Himanshu Arora
University of Miami
USA